Multi-Scale Modeling of Hippocampal Dynamics and Neural Prostheses
Theodore W. Berger, Ph.D.
Project Leader
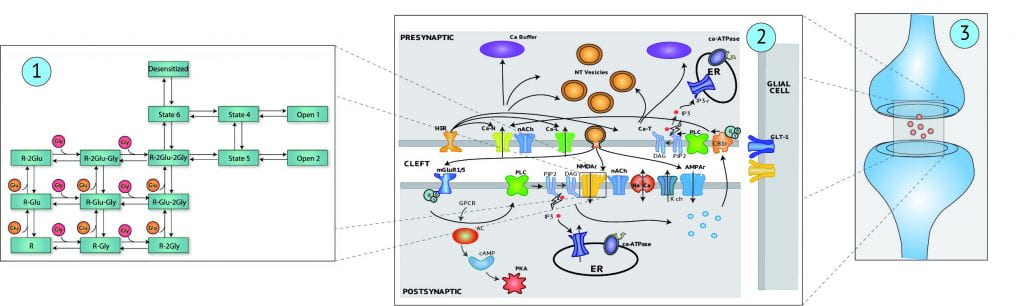
Neuroscience has reached a stage of development at which further understanding of complex systems, such as the hippocampus and other cortical regions that underlie cognition and higher thought processes, will depend on mathematical modeling as a means to organize experimental data that is known, and to systematically explore the unknown. The research objectives of Core Project #4 are to further develop and apply methodologies based on principles of nonlinear systems theory for experimentally-based, mathematical modeling of neurons and neural systems. This approach leads to what are commonly termed “non-parametric” or “input-output” models, which we feel are well-suited for the mammalian brain, because of its complexity, and the large number of unknowns. Parametric models, for which the parameters can be interpreted precisely with respect to underlying biological processes, have often been developed for hippocampus. Thus, we also will examine means for the synergistic use of non-parametric and parametric modeling methods.
- Input-output nonlinear modeling of hippocampus
- In-depth analyses of multi-input, multi-output (MIMO) models. There are several fundamental questions that must be addressed with respect to the existing MIMO data sets, including: (i) the apparent heterogeneity of the kernels recorded from the same animal during the same session. Differences in kernel functions could mean differences in feedback or feedforward connections with interneurons, differences in the density of voltage-dependent conductances, among other possibilities; (ii) correlations between classes of kernels functions and anatomical location within the hippocampus; and (iii) analyses of cross-kernels, having implications with respect to patterns of termination onto the postsynaptic dendritic tree.
- Control-theory based modeling of dentate-to-CA1. We will improve our single-input, single-output hippocampal CA3 prosthetic system using control theory. More specifically, we will build a dentate-CA1 output model and a inverse (output-input) CA1 model and use them to “drive” the CA1 region to its desired output trajectory following a control scheme.
- Multi-input, multi-output modeling of the hippocampal “trisynaptic pathway”. The hippocampus is composed of a ”trisynaptic pathway,” consisting of the entorhinal-to-dentate, dentate-to-CA3, and CA3-to-CA1 projections. We already have obtained the multi-input, multi-output model for the CA3-to-CA1 component of hippocampus. Using data provided by Dr. Sam Deadwyler from Wake Forest University, we also will obtain multi-input, multi-output models for the entorhinal-to-dentate and the dentate-to-CA3 components. This will provide the first ever quantitative description of the transformations that underlie the conversion of short-term memory to long-term memory.
- Multi-input, multi-output test for “cascade” vs. “feedforward” model of the hippocampus. In addition to the pathways described above, there is both anatomical and electrophysiological evidence for entorhinal-to-CA3 and entorhinal-to-CA1, implying a feedforward rather than a cascade model of hippocampal intrinsic circuitry. We will use our input-output models to test this hypothesis.
- Non-stationary aspects of hippocampal dynamics. These studies will focus on modeling the activity-dependent plasticity of the hippocampal dynamics.
- Characterize the time-evolution of multi-input, multi-output nonlinear dynamics during learning and memory formation. Using data recorded from naïve rats being trained to perform associative learning task, we will build time-varying multi-input, multi-output models for CA3-CA1 using non-stationary modeling techniques such as adaptive filter algorithms.
- Identify the “learning rules” underlying system non-stationarity. It is well established that certain forms of plasticity (long-term potentiation, or LTP) occur in response to specific input pattern, and that plasticity will in turn be manifest as a change in the systems’ input-output function. We will conduct simulation and mathematical analysis to explain the evolutions of the multi-input, multi-output CA3-CA1 dynamics as the consequences of the input flow and the learn rules.
- Expand the parametric model of the glutamatergic synapse to higher levels of complexity, both in the temporal and hierarchical scales. In addition to the non-parametric modeling issues described above, we also propose to develop new parametric models and modeling methods to expand our previous work on glutamatergic synapses, and the non-linear interactions between biomolecular pathways and electrophysiological activity.
- Increase the number of mechanisms modeled both pre- and postsynaptically. We propose to include additional elements and pathways to our parametric synaptic model, focusing more specifically on mechanisms that have not been implemented and have been established to participate in the regulation of synaptic efficacy.
- Investigate effects of synaptic activity on longer term biomolecular changes (e.g. plasticity) and their integration in neuronal spiking and neural network dynamics.
- Parametric vs non-parametric model comparisons. This work seeks to advance the notion that significant benefits can accrue from the combined (synergistic) use of the two modeling approaches in the study of single hippocampal neurons.
- Build non-parametric models of single hippocampal neurons. The models will be able to predict both subthreshold membrane potential and spike timing for broadband inputs.
- Build a realistic parametric model of single hippocampal neurons by combining presynaptic and post-synaptic parametric models. The presynaptic model refers to the residual calcium-based facilitation/depression model; the post-synaptic model is the morphologically realist compartmental model.
- Extract the functional input-output transformational property of the realistic parametric model by estimating its equivalent non-parametric model. This work will also elucidate how the key biological processes (represented as the key parameters of the realistic parametric model) determine the signal transmission of hippocampal neurons.
- Canonically test existing “reduced” parametric models. Many parametric neuron models have been developed for large-scale simulations which we call “reduced” because they include minimal numbers of parameters (to reduced computation time). These models were generally validated with limited input patterns. We will formally validate these models by comparing their non-parametric models with the non-parametric model of the “realistic” model.
- Non-parametrically validate and modify the realistic parametric model. Since the non-parametric model estimated from experimental data constitutes a canonical and complete representation of the system dynamics and is not restricted by any prior assumptions, it will be used as the “ground truth” to evaluate the realistic parametric model. Furthermore, the non-parametric model may suggest specific modifications in the structure of the parametric model to improve its predictive performance. The improved parametric model will provide in turn the requisite biological interpretability of the model components.
- EONS specific aim: EONS (Elementary Objects of the Nervous System) is the modeling software package developed and released by Core 4. It consists of a platform in which elements that comprise a single glutamatergic synapse (both pre- and postsynaptically) as well as the underlying synaptic geometry can be modified, hence providing great insight as to how these elements and their interactions shape synaptic transmission. We propose to create the next generation simulation platform by integrating EONS into a multiscale simulation platform capable of simulating a large number of synapses and a large number of neurons. This platform (MEMORY – Multiscale integrated mOdel of the nervous sYstem) constitutes a natural extension of EONS to answer multiscale scientific questions:
- Impact of synaptic molecular changes on neuronal spiking and network dynamics;
- Impact of electrical activity on longer term biomolecular dynamics and biochemical processes (e.g. second-messenger pathway activation);
We propose to develop the methodologies necessary for the implementation and simulation of this complex multiscale framework (computational methods, LoD problems, etc.). We also propose to extend our modeling work to a multi-node cluster environment, allowing us to launch simultaneously multiple simulations for faster parameter optimization and sensitivity analysis.
Selected Publications
Allam S.L., Bouteiller J-M.C., Hu E.Y., Ambert N., Greget R., Bischoff S., Baudry M., Berger T.W., Synaptic Efficacy as a Function of Ionotropic Receptor Distribution: A Computational Study, PLOS ONE 10(10):e0140333, 2015. [PMC4610697]
Hu E.Y., Bouteiller J-M.C., Song D., Baudry M., Berger T.W., Volterra representation enables modeling of complex synaptic nonlinear dynamics in large-scale simulations. Frontiers in Computational Neuroscience 9:112, 2015. [PMC4585022]
Allam S.L., Ghaderi V.S., Bouteiller J-M.C., Legendre A., Bischoff S., Baudry M., Berger T.W., A computational model to investigate astrocytic glutamate uptake influence on synaptic transmission and neuronal spiking, Frontiers in Computational Neuroscience 6(70), 2012. [PMC3461576]
Greget R., Pernot F., Bouteiller J-M. C., Ghaderi V., Allam S. L., Keller A. F., Ambert N., Sarmis M., Haeberle O., Faupel M., Bischoff S., Berger T. W., Baudry M. (2011), Simulation of Postsynaptic Glutamate Receptors Reveals Critical Features of Glutamatergic Transmission”, PLoS ONE 6(12): e28380, 2011. [PMC3240618]
Marmarelis V.Z., D.C. Shin, D. Song, R.E. Hampson, S.A. Deadwyler, and T.W. Berger. Nonlinear modeling of dynamic interactions within neuronal ensembles using Principal Dynamic Modes. Journal of Computational Neuroscience 34(1): 73-87, 2012. [PMC3531564]
Marmarelis V.Z., D.C. Shin, D. Song, R.E. Hampson, S.A. Deadwyler, and T.W. Berger. Design of optimal stimulation patterns for neuronal ensembles based on Volterra-type hierarchical modeling. Journal of Neural Engineering 9(6): 066003, 2012. [PMC3538881]
Hampson, R.E., G.A. Gerhardt, V.Z. Marmarelis, D. Song, I. Opris, L. Santos, T.W. Berger, and S.A. Deadwyler. Facilitation and restoration of cognitive function in primate prefrontal cortex by a neuroprosthesis that utilizes minicolumn-specific neural firing. Journal of Neural Engineering 9(6): 056012, 2012. [PMC3505670]
Berger T.W., D. Song, R.H.M. Chan, V.Z. Marmarelis, R.E. Hampson, S.A. Deadwyler, J. LaCoss, J. Wills, and J.J. Granacki. A hippocampal cognitive prosthesis: Multi-Input, Multi-Output nonlinear modeling and VLSI implementation. IEEE Transactions on Neural Systems & Rehabilitation Engineering 20(2):198-211, 2012. [PMC3395724]
Hampson, R.E., D. Song, R.H.M. Chan, A.J. Sweatt, M.R. Riley, A.V. Goonawardena, V.Z. Marmarelis, G.A. Gerhardt, T.W. Berger, and S.A. Deadwyler. Closing the loop for memory prostheses: Detecting the role of hippocampal neural ensembles using nonlinear models IEEE Transactions on Neural Systems & Rehabilitation Engineering 20(4):510-525, 2012. [PMC3395725]
Hampson R.E., D. Song, R.H.M. Chan, A.J. Sweatt, J. Fuqua, G.A. Gerhardt, D. Shin, V.Z. Marmarelis, T.W. Berger, and S.A. Deadwyle. A nonlinear model for hippocampal cognitive prosthesis: Memory facilitation by hippocampal ensemble stimulation. IEEE Transactions on Neural Systems & Rehabilitation Engineering 20(2):184-197, 2012. [PMC3397311]
Berger, T.W. R.E. Hampson, D. Song, A. Goonawardena, V.Z. Marmarelis, and S.A. Deadwyler. A cortical neural prosthesis for restoring and enhancing memory. Journal of Neural Engineering 55(2): 046017, 2011. [PDF]— [PMC3141091]
Berger, T.W. and Et Al. A cortical neural prosthesis for restoring and enhancing memory. Journal of Neural Engineering 55(2): 046017, 2011. [PDF]— [PMC3141091]
Lu, U., D. Song, and T.W. Berger. Nonlinear Dynamic Modeling of Synaptically Driven Single Hippocampal Neuron Intracellular Activity. Annual International Conference of the IEEE 58(5): 1303-1313, 2011. [PDF]— [PMC3125057]
Berger, T.W., D. Song, R.H.M. Chan, and V. Marmarelis. The neurobiological basis of cognition: Indentification by multi-input, multioutput nonlinear dynamic modeling. Proceedings of the IEEE 98(3): 356-374, 2010. [PDF]— [PMC2917774]
Ambert, N., R. Greget, O. Haeberle, S. Bischoff, T.W. Berger, J-M. Bouteiller, and M. Baudry. Computational studies of NMDA receptor: differential effects of neuronal activity on efficacy of competitive and non-competitive antagonists. Open Access Bioinformatics 2: 113-125, 2010. [PDF]— [PMC3092546]
Song, D., V. Marmarelis and T.W. Berger. Parametric and non-parametric modeling of short-term synaptic plasticity. Part I: computational study. Journal of Computational Neuroscience 26(1): 1-19, 2009. [PDF]— [PMC2770349]
Song, D., Z. Wang, V. Marmarelis and T.W. Berger. Parametric and non-parametric modeling of short-term synaptic plasticity. Part II: experimental study. Journal of Computational Neuroscience 26(1): 21-37, 2009. [PDF]— [PMC2749717]
Dimoka, A., S.H. Courellis, G. Gholmieh, V.Z. Marmarelis and T.W. Berger. Modeling the nonlinear properties of the in vitro hippocampal perforant path-dentate system using multi-electrode array technology. IEEE Transactions on Biomedical Engineering 55(2): 693-702, 2008. [PDF] — [PMC2749727]
Dimoka, A., S.H. Courellis, V.Z. Marmarelis and T.W. Berger. Modeling the nonlinear dynamic interactions of afferent pathways in the dentate gyrus of the hippocampus. Annals of Biomedical Engineering 36(5): 852-864, 2008.[PDF] — [PMC2749714]
Kim, M., W. Soussou, G. Gholmieh, A. Ahuja, A.R. Tanguay Jr., T.W. Berger and R.D. Brinton. 17β-estradiol potentiates field excitatory postsynaptic potentials within each subfield of the hippocampus with greatest potentiation of the associational/commissural afferents of CA3. Neuroscience 141:391-406, 2006. [PDF]
Gholmieh, G., W. Soussou, M. Han, A. Ahuja, M.C. Hsiao, D. Song, A.R. Tanguay Jr. and T.W. Berger. Custom-designed, high-density conformal planar multielectrode arrays for brain slice electrophysiology. Journal of Neuroscience Methods 152:116-129, 2006. [PDF]
Berger, T.W., and Glanzman, D.L. Toward Replacement Parts for the Brain: Implantable Biomimetic Electronics as the Next Era in Neural Prosthetics. Cambridge, MA: MIT Press, 2005.
Gholmieh, G., S. Courellis, A. Dimoka, J.J. Granacki, V.Z. Marmarelis and T.W. Berger. An algorithm for real-time extraction of population EPSPs and spikes from hippocampal field potentials. Journal of Neuroscience Methods 136(2):111-121, 2004.[PDF]
Namarvar, H.H. and T.W. Berger. An efficient training algorithm for dynamic synapse neural networks using trust region methods. Neural Networks 16:585-591, 2003.
Gholmieh, G., S. Courellis, S. Fakheri, E. Cheung, V.Z. Marmarelis, M.Baudry and T.W. Berger. Detection and classification of neurotoxins using a novel short-term plasticity quantification method. Biosensors and Bioelectronics 18(12):1467-1478, 2003.[PDF]
Berger, T.W., J.J. Granacki, v.Z. Marmarelis, B.J. Sheu and A.R. Tanguay, Jr. Brain-implantable biomimetic electronics as neural prosthetics. Proceedings of the 1st International IEEE EMBS Conference on Neural Engineering, 108-111, 2003.
Song, D., Z. Wang, V.Z. Marmarelis, and T.W. Berger. Non-parametric interpretation and validation of parametric models of short-term plasticity. Proceedings of the 25th IEEE-EMBS Conference, 1901-1904, 2003.
Gholmieh, G., S.H. Courellis, D. Song, Z. Wang, V.Z. Marmarelis and Berger. Characterization of short-term plasticity of the dentate gyrus-CA3 system using nonlinear systems analysis. Proceedings of the 25th IEEE-EMBS Conference, 1929-1932, 2003.
Dimoka, A., S.H. Courellis, D. Song, V.Z. Marmarelis, and T.W. Berger. Identification of lateral and medial perforant path using single- and dual-input random impulse train stimulation. Proceedings of the 25th IEEE-EMBS Conference, 1933-1936, 2003.
Song, D., Z. Wang and T.W. Berger. Contribution of T-Type VDCC to TEA-Induced long-term synaptic modification in hippocampal CA1 and dentate gyrus. Hippocampus 12:689-697, 2002. [PDF]
Wang, Z., D. Song and T.W. Berger. Contribution of NMDA receptor channels to the expression of LTP in the hippocampal dentate gyrus. Hippocampus 12:680-688, 2002. [PDF]
Song, D., X. Xiaping, Z. Wang and T.W. Berger. Differential effect of TEA on long-term synaptic modification in hippocampal CA1 and Dentate Gyrus in vitro. Neurobiology of Learning and Memory 76:375-387, 2001. [PDF]
Alataris, K., T.W. Berger and V.Z. Marmarelis. A novel network for nonlinear modeling of neural systems with arbitrary point-process inputs. Neural Networks 13(2):255-266, 2000. [PDF]
Foy, M., T.W. Berger and R.F. Thompson. Estrogen and neural plasticity. Current Direction in Psychological Science 9(5):148-152, 2000. [PDF]
Chian, M., V.Z. Marmarelis and T.W. Berger. Decomposition of neural systems with nonlinear feedback using stimulus-response data. Neurocomputing 26-27:641-654, 1999. [PDF]
Liaw, J.-S. and T.W. Berger. Dynamic synapse: Harnessing the computing power of synaptic dynamics. Neurocomputing 26-27:199-206, 1999.
Iatrou, M., T.W. Berger and V.Z. Marmarelis. Modeling of nonlinear nonstationay dynamic systems with a novel class of artificial neural networks. IEEE Trans. Neural Networks, 10:327-339, 1999.
Yeckel, M.F. and T.W. Berger. Spatial distribution of potentiated synapses in hippocampus: Dependence on cellular mechanisms and network properties. J. Neuroscience, 18:438-450, 1998.
Tsai, R.H., J.B. Sheu and T.W. Berger. A VLSI neural network processor based on a model of the hippocampus. Analog Integrated Circuits & Signal Processing 15:201-213, 1998.
Xie, X., J.-S. Liaw, M. Baudry and T.W. Berger. Novel expression mechanism for synaptic potentiation: Alignment of presynaptic release site and postsynaptic receptor. Proc. National Academy of Sciences 94:6983-6988, 1997. [PMC21271]
Liaw, J.-S., and T.W. Berger. The dynamic Synapse: A new concept for neural representation and computation. Hippocampus, 6:591-600, 1996.
Berger, T.W., B.J. Sheu, and R.H.-K. Tsai. Biologically realistic models of memory function implemented using analog VLSI technology. In: Microsystems Technology for Multimedia Applications, B. Sheu, M. Ismail, E. Sanchez-Sinencio and T. Wu (Eds.). N.Y.:IEEE Press, pp. 381-394, 1995.
Yeckel, M.Y. and T.W. Berger. Monosynaptic excitation of CA1 hippocampal pyramidal neurons by afferents from the entorhinal cortex. Hippocampus 5(2):108-114, 1995.